L’intelligence artificielle et l’analyse prédictive ouvrent une ère nouvelle pour la santé mentale, permettant une anticipation proactive des crises et des traitements sur mesure. Si ces technologies offrent des perspectives prometteuses, elles soulèvent aussi des défis liés à l’exactitude des modèles, à la protection des données et à leur intégration clinique. Une exploration des enjeux et des opportunités de cette révolution en marche.
Ces dernières années, la santé mentale a connu des avancées majeures, en grande partie grâce à l’introduction de l’intelligence artificielle (IA) et de l’analyse prédictive. Cette technologie révolutionne la manière dont les professionnels identifient, surveillent et interviennent dans les troubles mentaux. En exploitant d’énormes volumes de données et en détectant des schémas subtils, l’IA ouvre de nouvelles perspectives pour anticiper les crises, personnaliser les traitements et améliorer les soins de manière significative.
L’analyse prédictive : anticiper les crises pour mieux intervenir
L’une des applications les plus prometteuses de l’IA en santé mentale réside dans sa capacité à prévoir des crises avant qu’elles ne surviennent. L’analyse prédictive repose sur des données en temps réel collectées via des dispositifs connectés, tels que des montres intelligentes ou des applications mobiles, pour surveiller divers indicateurs comportementaux et physiologiques. Parmi ces signaux, on trouve :
- Les variations de la fréquence cardiaque.
- La qualité et les perturbations du sommeil.
- Les changements dans les niveaux d’activité physique.
- La diminution des interactions sociales.
Par exemple, une diminution notable de la communication sociale ou des habitudes de sommeil altérées peut indiquer un risque accru d’épisodes dépressifs ou anxieux. En détectant ces signaux avant qu’ils ne deviennent critiques, les professionnels de santé mentale peuvent intervenir proactivement, offrant un soutien avant que la situation ne dégénère.
Les défis de l’analyse prédictive en santé mentale
Bien que prometteuse, l’analyse prédictive dans ce domaine soulève plusieurs défis :
Exactitude et biais des algorithmes :
Les modèles d’IA doivent être capables de fournir des prédictions fiables pour différentes populations. Une attention particulière doit être accordée aux biais potentiels des ensembles de données, afin de garantir que les résultats soient pertinents pour des groupes sous-représentés ou présentant des caractéristiques atypiques.
Collaboration interdisciplinaire :
Les data scientists doivent travailler étroitement avec des psychologues, psychiatres et autres experts pour s’assurer que les modèles sont non seulement statistiquement valides, mais également cliniquement utiles. Cette collaboration permet d’intégrer les résultats dans des pratiques cliniques adaptées.
Éthique et confidentialité des données :
L’utilisation de données sensibles, comme celles collectées via des appareils connectés, nécessite des protocoles stricts pour protéger la vie privée des patients et éviter toute utilisation abusive des informations.
Intégration dans les processus cliniques :
Les systèmes d’analyse prédictive doivent être conçus pour s’intégrer aux flux de travail des professionnels de santé, afin que les résultats soient interprétés dans leur contexte et utilisés pour prendre des décisions éclairées.
Vers une personnalisation des soins grâce à l’analyse prédictive
En combinant des données de santé comportementale, physiologique et sociale, l’analyse prédictive permet d’élaborer des plans de traitement personnalisés. Ces approches augmentent l’efficacité des soins en adaptant les interventions aux besoins spécifiques de chaque individu. Par exemple, un patient identifié comme étant à risque d’une crise d’anxiété pourrait recevoir des alertes sur son smartphone, accompagnées de recommandations personnalisées pour gérer ses symptômes, comme des exercices de respiration ou des suggestions d’activités apaisantes.
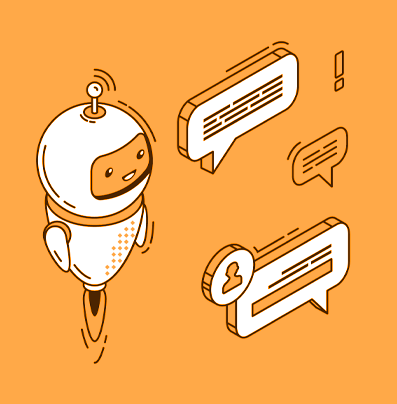
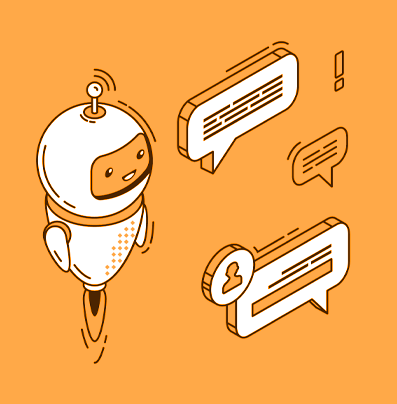
démonstration
EN LIGNE
démonstration EN LIGNE
Le 14 Mai 2025
à partir de 18h30
Venez découvrir Lact Assistance IA+ et rencontrer nos experts avec une démonstration en direct
Un potentiel transformateur pour l’avenir de la santé mentale
L’analyse prédictive représente un tournant majeur dans la santé mentale, en rendant possible une approche proactive et individualisée des soins. Cependant, son déploiement doit s’accompagner de garanties éthiques et techniques solides, afin que son impact soit réellement bénéfique pour tous les patients. En surmontant les défis actuels et en favorisant une collaboration étroite entre experts techniques et cliniques, cette technologie a le potentiel de transformer durablement le paysage de la santé mentale.
Un outil incontournable pour les mutuelles
Développée par le centre de recherche français LACT, LACT Assistance IA+ est un outil de pointe qui permet d’identifier, de prévenir et de prendre en charge les problématiques psychologiques et relationnelles des adhérents. Ce dispositif novateur repose sur une intelligence artificielle conversationnelle, conçue selon les principes du diagnostic systémique et de l’intervention stratégique, garantissant un accompagnement rapide, personnalisé et sécurisé.
Prévenir les risques avant qu’ils ne deviennent critiques : Avec un accès 24h/24 et 7j/7, LACT Assistance IA+ offre aux adhérents un soutien immédiat et confidentiel. Cette réactivité permet d’intervenir précocement face aux premiers signaux de stress, de mal-être ou de conflits relationnels, réduisant ainsi le risque de complications et d’absentéisme.
Une prise en charge humaine pour les situations complexes : Lorsqu’une intervention plus approfondie est nécessaire, LACT Assistance IA+ oriente les adhérents vers des consultations avec des psychologues ou des coachs certifiés, spécialisés dans la gestion des risques psychosociaux. Cette complémentarité garantit une prise en charge globale et efficace, adaptée aux besoins de chacun.
Des données anonymisées pour orienter vos actions de prévention : Grâce à son système d’analyse, LACT Assistance IA+ fournit des indicateurs anonymisés sur les problématiques récurrentes et émergentes au sein des populations couvertes. Ces données permettent de cibler vos actions de prévention et d’ajuster vos stratégies pour répondre aux besoins, tout en respectant strictement la confidentialité des échanges.
Des bénéfices concrets pour les adhérents des mutuelles : LACT Assistance IA+ permet de réduire les coûts associés aux risques psychosociaux, tout en valorisant l’image des mutuelles comme acteurs innovants et engagés. Les adhérents bénéficient d’un accès simple et sécurisé à des outils et des professionnels capables de les accompagner face aux défis de la vie personnelle et professionnelle.
Les retours des utilisateurs soulignent l’impact positif de cette approche. Comme en témoigne Isabelle, responsable chez un partenaire : "Avec LACT Assistance IA+, nous avons pu structurer nos actions de prévention des risques psychosociaux. Les indicateurs fournis nous permettent d’agir avec précision, et les adhérents apprécient la qualité du soutien proposé." Claire, adhérente, ajoute : "J’ai trouvé un appui précieux dans une période difficile, grâce à un accompagnement à la fois rapide et rassurant."
Entièrement conforme aux exigences HDS sur la protection des données de santé, LACT Assistance IA+ constitue une réponse efficace aux enjeux de prévention et d’accompagnement des risques psychosociaux.
Bibliographie
- The Pan-European Mental Health Coalition. WHO. https://www.euro.who.int/en/health-topics/health-policy/european -programme-of-work/flagship-initiatives/the-pan-european-mental-health-coalition [accessed 2023-01-23]
- WHO Regional Office for Europe. European Programme of Work, 2020–2025. WHO. 2020. URL: https://www.who.int/europe/about-us/our-work/european-programme-of-work [accessed 2023-01-23]
- Reddy S, Fox J, Purohit MP. Artificial intelligence-enabled healthcare delivery. J R Soc Med 2019 Jan;112(1):22-28 [FREE Full text] [CrossRef] [Medline]
- He J, Baxter SL, Xu J, Xu J, Zhou X, Zhang K. The practical implementation of artificial intelligence technologies in medicine. Nat Med 2019 Jan;25(1):30-36 [FREE Full text] [CrossRef] [Medline]
- Borges do Nascimento IJ, Marcolino MS, Abdulazeem HM, Weerasekara I, Azzopardi-Muscat N, Gonçalves MA, et al. Impact of Big Data Analytics on People's Health: Overview of Systematic Reviews and Recommendations for Future Studies. J Med Internet Res 2021 Apr 13;23(4):e27275 [FREE Full text] [CrossRef] [Medline]
- Andaur Navarro CL, Damen JAA, Takada T, Nijman SWJ, Dhiman P, Ma J, et al. Risk of bias in studies on prediction models developed using supervised machine learning techniques: systematic review. BMJ 2021 Oct 20;375:n2281 [FREE Full text] [CrossRef] [Medline]
- Felzmann H, Fosch-Villaronga E, Lutz C, Tamò-Larrieux A. Towards Transparency by Design for Artificial Intelligence. Sci Eng Ethics 2020 Dec 16;26(6):3333-3361 [FREE Full text] [CrossRef] [Medline]
- Vollmer S, Mateen BA, Bohner G, Király FJ, Ghani R, Jonsson P, et al. Machine learning and artificial intelligence research for patient benefit: 20 critical questions on transparency, replicability, ethics, and effectiveness. BMJ 2020 Mar 20;368:l6927 [FREE Full text] [CrossRef] [Medline]
- Page MJ, McKenzie JE, Bossuyt PM, Boutron I, Hoffmann TC, Mulrow CD, et al. The PRISMA 2020 statement: An updated guideline for reporting systematic reviews. Int J Surg 2021 Apr;88:105906. [CrossRef] [Medline]
- Moons KGM, de Groot JAH, Bouwmeester W, Vergouwe Y, Mallett S, Altman DG, et al. Critical appraisal and data extraction for systematic reviews of prediction modelling studies: the CHARMS checklist. PLoS Med 2014 Oct 14;11(10):e1001744 [FREE Full text] [CrossRef] [Medline]
- Higgins JPT, Savović J, Page MJ, Elbers RG, Sterne JAC. Chapter 8: Assessing risk of bias in a randomized trial. In: Higgins JPT, Thomas J, Chandler J, Cumpston M, Li T, Page MJ, et al, editors. Cochrane Handbook for Systematic Reviews of Interventions version 6.3 (updated February 2022). London, UK: Cochrane; 2022.
- Wolff RF, Moons KG, Riley RD, Whiting PF, Westwood M, Collins GS, et al. PROBAST: A Tool to Assess the Risk of Bias and Applicability of Prediction Model Studies. Ann Intern Med 2019 Jan 01;170(1):51. [CrossRef]
- Higgins J. Cochrane Handbook for Systematic Reviews of Interventions Version 5. The Cochrane Collaboration. London, UK: Cochrane Collaboration; 2011. URL: https://training.cochrane.org/handbook [accessed 2023-01-24]
- Caye A, Agnew-Blais J, Arseneault L, Gonçalves H, Kieling C, Langley K, et al. A risk calculator to predict adult attention-deficit/hyperactivity disorder: generation and external validation in three birth cohorts and one clinical sample. Epidemiol Psychiatr Sci 2019 May 15;29:e37. [CrossRef]
- de Pierrefeu A, Löfstedt T, Laidi C, Hadj-Selem F, Bourgin J, Hajek T, et al. Identifying a neuroanatomical signature of schizophrenia, reproducible across sites and stages, using machine learning with structured sparsity. Acta Psychiatr Scand 2018 Dec 21;138(6):571-580. [CrossRef] [Medline]
- Vieira S, Gong QY, Pinaya WHL, Scarpazza C, Tognin S, Crespo-Facorro B, et al. Using Machine Learning and Structural Neuroimaging to Detect First Episode Psychosis: Reconsidering the Evidence. Schizophr Bull 2020 Jan 04;46(1):17-26 [FREE Full text] [CrossRef] [Medline]
- Bae Y, Kumarasamy K, Ali IM, Korfiatis P, Akkus Z, Erickson BJ. Differences Between Schizophrenic and Normal Subjects Using Network Properties from fMRI. J Digit Imaging 2018 Apr 18;31(2):252-261 [FREE Full text] [CrossRef] [Medline]
- Chand GB, Dwyer DB, Erus G, Sotiras A, Varol E, Srinivasan D, et al. Two distinct neuroanatomical subtypes of schizophrenia revealed using machine learning. Brain 2020 Mar 01;143(3):1027-1038 [FREE Full text] [CrossRef] [Medline]
- Koutsouleris N, Wobrock T, Guse B, Langguth B, Landgrebe M, Eichhammer P, et al. Predicting Response to Repetitive Transcranial Magnetic Stimulation in Patients With Schizophrenia Using Structural Magnetic Resonance Imaging: A Multisite Machine Learning Analysis. Schizophr Bull 2018 Aug 20;44(5):1021-1034 [FREE Full text] [CrossRef] [Medline]
- Anderson JP, Icten Z, Alas V, Benson C, Joshi K. Comparison and predictors of treatment adherence and remission among patients with schizophrenia treated with paliperidone palmitate or atypical oral antipsychotics in community behavioral health organizations. BMC Psychiatry 2017 Oct 18;17(1):346 [FREE Full text] [CrossRef] [Medline]
- Chung Y, Addington J, Bearden CE, Cadenhead K, Cornblatt B, Mathalon DH, North American Prodrome Longitudinal Study (NAPLS) Consortiumthe Pediatric Imaging‚ Neurocognition‚Genetics (PING) Study Consortium. Use of Machine Learning to Determine Deviance in Neuroanatomical Maturity Associated With Future Psychosis in Youths at Clinically High Risk. JAMA Psychiatry 2018 Sep 01;75(9):960-968 [FREE Full text] [CrossRef] [Medline]
- Dillon K, Calhoun V, Wang Y. A robust sparse-modeling framework for estimating schizophrenia biomarkers from fMRI. J Neurosci Methods 2017 Jan 30;276:46-55 [FREE Full text] [CrossRef] [Medline]
- Schreiner M, Forsyth JK, Karlsgodt KH, Anderson AE, Hirsh N, Kushan L, et al. Intrinsic Connectivity Network-Based Classification and Detection of Psychotic Symptoms in Youth With 22q11.2 Deletions. Cereb Cortex 2017 Jun 01;27(6):3294-3306 [FREE Full text] [CrossRef] [Medline]
- Lefort-Besnard J, Varoquaux G, Derntl B, Gruber O, Aleman A, Jardri R, et al. Patterns of schizophrenia symptoms: hidden structure in the PANSS questionnaire. Transl Psychiatry 2018 Oct 30;8(1):237 [FREE Full text] [CrossRef] [Medline]
- Brodey B, Girgis R, Favorov O, Bearden C, Woods S, Addington J, et al. The Early Psychosis Screener for Internet (EPSI)-SR: Predicting 12 month psychotic conversion using machine learning. Schizophr Res 2019 Jun;208:390-396 [FREE Full text] [CrossRef] [Medline]
- Koutsouleris N, Kahn RS, Chekroud AM, Leucht S, Falkai P, Wobrock T, et al. Multisite prediction of 4-week and 52-week treatment outcomes in patients with first-episode psychosis: a machine learning approach. The Lancet Psychiatry 2016 Oct;3(10):935-946. [CrossRef]
- Nieuwenhuis M, Schnack HG, van Haren NE, Lappin J, Morgan C, Reinders AA, et al. Multi-center MRI prediction models: Predicting sex and illness course in first episode psychosis patients. Neuroimage 2017 Jan 15;145(Pt B):246-253 [FREE Full text] [CrossRef] [Medline]
- Rozycki M, Satterthwaite TD, Koutsouleris N, Erus G, Doshi J, Wolf DH, et al. Multisite Machine Learning Analysis Provides a Robust Structural Imaging Signature of Schizophrenia Detectable Across Diverse Patient Populations and Within Individuals. Schizophr Bull 2018 Aug 20;44(5):1035-1044 [FREE Full text] [CrossRef] [Medline]
- Chen J, Zang Z, Braun U, Schwarz K, Harneit A, Kremer T, et al. Association of a Reproducible Epigenetic Risk Profile for Schizophrenia With Brain Methylation and Function. JAMA Psychiatry 2020 Jun 01;77(6):628-636 [FREE Full text] [CrossRef] [Medline]
- Fond G, Bulzacka E, Boucekine M, Schürhoff F, Berna F, Godin O, FACE-SZ (FondaMental Academic Centers of Expertise for Schizophrenia) group, et al. Machine learning for predicting psychotic relapse at 2 years in schizophrenia in the national FACE-SZ cohort. Prog Neuropsychopharmacol Biol Psychiatry 2019 Jun 08;92:8-18. [CrossRef] [Medline]
- Gibbons RD, Chattopadhyay I, Meltzer HY, Kane JM, Guinart D. Development of a computerized adaptive diagnostic screening tool for psychosis. Schizophr Res 2022 Jul;245:116-121. [CrossRef] [Medline]
- Martinuzzi E, Barbosa S, Daoudlarian D, Bel Haj Ali W, Gilet C, Fillatre L, OPTiMiSE Study Group. Stratification and prediction of remission in first-episode psychosis patients: the OPTiMiSE cohort study. Transl Psychiatry 2019 Jan 17;9(1):20 [FREE Full text] [CrossRef] [Medline]
- Skåtun KC, Kaufmann T, Doan NT, Alnæs D, Córdova-Palomera A, Jönsson EG, KaSP, et al. Consistent Functional Connectivity Alterations in Schizophrenia Spectrum Disorder: A Multisite Study. Schizophr Bull 2017 Jul 01;43(4):914-924 [FREE Full text] [CrossRef] [Medline]
- Busk J, Faurholt-Jepsen M, Frost M, Bardram JE, Vedel Kessing L, Winther O. Forecasting Mood in Bipolar Disorder From Smartphone Self-assessments: Hierarchical Bayesian Approach. JMIR Mhealth Uhealth 2020 Apr 01;8(4):e15028 [FREE Full text] [CrossRef] [Medline]
- Sun ZY, Houenou J, Duclap D, Sarrazin S, Linke J, Daban C, et al. Shape analysis of the cingulum, uncinate and arcuate fasciculi in patients with bipolar disorder. J Psychiatry Neurosci 2017 Jan;42(1):27-36 [FREE Full text] [CrossRef] [Medline]
- Kim TT, Dufour S, Xu C, Cohen ZD, Sylvia L, Deckersbach T, et al. Predictive modeling for response to lithium and quetiapine in bipolar disorder. Bipolar Disord 2019 Aug;21(5):428-436. [CrossRef] [Medline]
- Lei D, Li W, Tallman MJ, Patino LR, McNamara RK, Strawn JR, et al. Changes in the brain structural connectome after a prospective randomized clinical trial of lithium and quetiapine treatment in youth with bipolar disorder. Neuropsychopharmacology 2021 Jun;46(7):1315-1323 [FREE Full text] [CrossRef] [Medline]
- Brown EC, Clark DL, Forkert ND, Molnar CP, Kiss ZHT, Ramasubbu R. Metabolic activity in subcallosal cingulate predicts response to deep brain stimulation for depression. Neuropsychopharmacology 2020 Sep;45(10):1681-1688 [FREE Full text] [CrossRef] [Medline]
- Carrillo F, Sigman M, Fernández Slezak D, Ashton P, Fitzgerald L, Stroud J, et al. Natural speech algorithm applied to baseline interview data can predict which patients will respond to psilocybin for treatment-resistant depression. J Affect Disord 2018 Apr 01;230:84-86. [CrossRef] [Medline]
- Sikora M, Heffernan J, Avery ET, Mickey BJ, Zubieta J, Peciña M. Salience Network Functional Connectivity Predicts Placebo Effects in Major Depression. Biol Psychiatry Cogn Neurosci Neuroimaging 2016 Jan;1(1):68-76 [FREE Full text] [CrossRef] [Medline]
- Al-Kaysi AM, Al-Ani A, Loo CK, Powell TY, Martin DM, Breakspear M, et al. Predicting tDCS treatment outcomes of patients with major depressive disorder using automated EEG classification. J Affect Disord 2017 Jan 15;208:597-603. [CrossRef] [Medline]
- Athreya A, Iyer R, Neavin D, Wang L, Weinshilboum R, Kaddurah-Daouk R, et al. Augmentation of Physician Assessments with Multi-Omics Enhances Predictability of Drug Response: A Case Study of Major Depressive Disorder. IEEE Comput Intell Mag 2018 Aug;13(3):20-31. [CrossRef]
- Bai R, Xiao L, Guo Y, Zhu X, Li N, Wang Y, et al. Tracking and Monitoring Mood Stability of Patients With Major Depressive Disorder by Machine Learning Models Using Passive Digital Data: Prospective Naturalistic Multicenter Study. JMIR Mhealth Uhealth 2021 Mar 08;9(3):e24365 [FREE Full text] [CrossRef] [Medline]
- Bao Z, Zhao X, Li J, Zhang G, Wu H, Ning Y, et al. Prediction of repeated-dose intravenous ketamine response in major depressive disorder using the GWAS-based machine learning approach. J Psychiatr Res 2021 Jun;138:284-290. [CrossRef] [Medline]
- Bartlett EA, DeLorenzo C, Sharma P, Yang J, Zhang M, Petkova E, et al. Pretreatment and early-treatment cortical thickness is associated with SSRI treatment response in major depressive disorder. Neuropsychopharmacology 2018 Oct 19;43(11):2221-2230 [FREE Full text] [CrossRef] [Medline]
- Bremer V, Becker D, Kolovos S, Funk B, van Breda W, Hoogendoorn M, et al. Predicting Therapy Success and Costs for Personalized Treatment Recommendations Using Baseline Characteristics: Data-Driven Analysis. J Med Internet Res 2018 Aug 21;20(8):e10275 [FREE Full text] [CrossRef] [Medline]
- Goerigk S, Hilbert S, Jobst A, Falkai P, Bühner M, Stachl C, et al. Predicting instructed simulation and dissimulation when screening for depressive symptoms. Eur Arch Psychiatry Clin Neurosci 2020 Mar 12;270(2):153-168. [CrossRef] [Medline]
- Hopman H, Chan S, Chu W, Lu H, Tse C, Chau S, et al. Personalized prediction of transcranial magnetic stimulation clinical response in patients with treatment-refractory depression using neuroimaging biomarkers and machine learning. J Affect Disord 2021 Jul 01;290:261-271. [CrossRef] [Medline]
- Monaro M, Toncini A, Ferracuti S, Tessari G, Vaccaro MG, De Fazio P, et al. The Detection of Malingering: A New Tool to Identify Made-Up Depression. Front Psychiatry 2018 Jun 8;9:249 [FREE Full text] [CrossRef] [Medline]
- Nie Z, Vairavan S, Narayan VA, Ye J, Li QS. Predictive modeling of treatment resistant depression using data from STARD and an independent clinical study. PLoS One 2018 Jun 7;13(6):e0197268 [FREE Full text] [CrossRef] [Medline]
- Setoyama D, Kato TA, Hashimoto R, Kunugi H, Hattori K, Hayakawa K, et al. Plasma Metabolites Predict Severity of Depression and Suicidal Ideation in Psychiatric Patients-A Multicenter Pilot Analysis. PLoS One 2016 Dec 16;11(12):e0165267 [FREE Full text] [CrossRef] [Medline]
- Shepherd-Banigan M, Smith VA, Lindquist JH, Cary MP, Miller KEM, Chapman JG, et al. Identifying treatment effects of an informal caregiver education intervention to increase days in the community and decrease caregiver distress: a machine-learning secondary analysis of subgroup effects in the HI-FIVES randomized clinical trial. Trials 2020 Feb 14;21(1):189 [FREE Full text] [CrossRef] [Medline]
- Zhao M, Feng Z. Machine Learning Methods to Evaluate the Depression Status of Chinese Recruits: A Diagnostic Study. NDT 2020 Nov;16:2743-2752. [CrossRef]
- Schultebraucks K, Choi KW, Galatzer-Levy IR, Bonanno GA. Discriminating Heterogeneous Trajectories of Resilience and Depression After Major Life Stressors Using Polygenic Scores. JAMA Psychiatry 2021 Jul 01;78(7):744-752 [FREE Full text] [CrossRef] [Medline]
- Castellani B, Griffiths F, Rajaram R, Gunn J. Exploring comorbid depression and physical health trajectories: A case-based computational modelling approach. J Eval Clin Pract 2018 Dec 02;24(6):1293-1309. [CrossRef] [Medline]
- Jacobson NC, Nemesure MD. Using Artificial Intelligence to Predict Change in Depression and Anxiety Symptoms in a Digital Intervention: Evidence from a Transdiagnostic Randomized Controlled Trial. Psychiatry Res 2021 Jan;295:113618 [FREE Full text] [CrossRef] [Medline]
- Kessler RC, Furukawa TA, Kato T, Luedtke A, Petukhova M, Sadikova E, et al. An individualized treatment rule to optimize probability of remission by continuation, switching, or combining antidepressant medications after failing a first-line antidepressant in a two-stage randomized trial. Psychol Med 2021 Mar 08;52(15):3371-3380. [CrossRef]
- Bondar J, Caye A, Chekroud AM, Kieling C. Symptom clusters in adolescent depression and differential response to treatment: a secondary analysis of the Treatment for Adolescents with Depression Study randomised trial. The Lancet Psychiatry 2020 Apr;7(4):337-343. [CrossRef]
- Kato M, Asami Y, Wajsbrot DB, Wang X, Boucher M, Prieto R, et al. Clustering patients by depression symptoms to predict venlafaxine ER antidepressant efficacy: Individual patient data analysis. J Psychiatr Res 2020 Oct;129:160-167 [FREE Full text] [CrossRef] [Medline]
- Bueno ML, Hommersom A, Lucas PJ, Janzing J. A probabilistic framework for predicting disease dynamics: A case study of psychotic depression. J Biomed Inform 2019 Jul;95:103232 [FREE Full text] [CrossRef] [Medline]
- Alexopoulos GS, Raue PJ, Banerjee S, Mauer E, Marino P, Soliman M, et al. Modifiable predictors of suicidal ideation during psychotherapy for late-life major depression. A machine learning approach. Transl Psychiatry 2021 Oct 18;11(1):536 [FREE Full text] [CrossRef] [Medline]
- Solomonov N, Lee J, Banerjee S, Flückiger C, Kanellopoulos D, Gunning FM, et al. Modifiable predictors of nonresponse to psychotherapies for late-life depression with executive dysfunction: a machine learning approach. Mol Psychiatry 2021 Sep 10;26(9):5190-5198 [FREE Full text] [CrossRef] [Medline]
- Bruijniks SJ, DeRubeis RJ, Lemmens LH, Peeters FP, Cuijpers P, Huibers MJ. The relation between therapy quality, therapy processes and outcomes and identifying for whom therapy quality matters in CBT and IPT for depression. Behav Res Ther 2021 Jan 28;139:103815. [CrossRef] [Medline]
- Di Y, Wang J, Li W, Zhu T. Using i-vectors from voice features to identify major depressive disorder. J Affect Disord 2021 Jun 01;288:161-166. [CrossRef] [Medline]
- Gunlicks-Stoessel M, Klimes-Dougan B, VanZomeren A, Ma S. Developing a data-driven algorithm for guiding selection between cognitive behavioral therapy, fluoxetine, and combination treatment for adolescent depression. Transl Psychiatry 2020 Sep 21;10(1):321 [FREE Full text] [CrossRef] [Medline]
- Iniesta R, Malki K, Maier W, Rietschel M, Mors O, Hauser J, et al. Combining clinical variables to optimize prediction of antidepressant treatment outcomes. J Psychiatr Res 2016 Jul;78:94-102 [FREE Full text] [CrossRef] [Medline]
- Liu Y, Admon R, Mellem MS, Belleau EL, Kaiser RH, Clegg R, et al. Machine Learning Identifies Large-Scale Reward-Related Activity Modulated by Dopaminergic Enhancement in Major Depression. Biological Psychiatry: Cognitive Neuroscience and Neuroimaging 2020 Feb;5(2):163-172. [CrossRef]
- Maglanoc LA, Kaufmann T, Jonassen R, Hilland E, Beck D, Landrø NI, et al. Multimodal fusion of structural and functional brain imaging in depression using linked independent component analysis. Hum Brain Mapp 2020 Jan;41(1):241-255 [FREE Full text] [CrossRef] [Medline]
- Mennen AC, Turk-Browne NB, Wallace G, Seok D, Jaganjac A, Stock J, et al. Cloud-Based Functional Magnetic Resonance Imaging Neurofeedback to Reduce the Negative Attentional Bias in Depression: A Proof-of-Concept Study. Biol Psychiatry Cogn Neurosci Neuroimaging 2021 Apr;6(4):490-497 [FREE Full text] [CrossRef] [Medline]
- Salvetat N, Van der Laan S, Vire B, Chimienti F, Cleophax S, Bronowicki JP, et al. RNA editing blood biomarkers for predicting mood alterations in HCV patients. J Neurovirol 2019 Dec 22;25(6):825-836 [FREE Full text] [CrossRef] [Medline]
- van Bronswijk SC, DeRubeis RJ, Lemmens LHJM, Peeters FPML, Keefe JR, Cohen ZD, et al. Precision medicine for long-term depression outcomes using the Personalized Advantage Index approach: cognitive therapy or interpersonal psychotherapy? Psychol. Med 2019 Nov 22;51(2):279-289. [CrossRef]
- van Bronswijk SC, Bruijniks SJE, Lorenzo-Luaces L, Derubeis RJ, Lemmens LHJM, Peeters FPML, et al. Cross-trial prediction in psychotherapy: External validation of the Personalized Advantage Index using machine learning in two Dutch randomized trials comparing CBT versus IPT for depression. Psychother Res 2021 Jan 23;31(1):78-91. [CrossRef] [Medline]
- Webb CA, Trivedi MH, Cohen ZD, Dillon DG, Fournier JC, Goer F, et al. Personalized prediction of antidepressant v. placebo response: evidence from the EMBARC study. Psychol Med 2018 Jul 2;49(07):1118-1127. [CrossRef]
- Wu W, Zhang Y, Jiang J, Lucas MV, Fonzo GA, Rolle CE, et al. An electroencephalographic signature predicts antidepressant response in major depression. Nat Biotechnol 2020 Apr 10;38(4):439-447 [FREE Full text] [CrossRef] [Medline]
- Fonzo GA, Etkin A, Zhang Y, Wu W, Cooper C, Chin-Fatt C, et al. Brain regulation of emotional conflict predicts antidepressant treatment response for depression. Nat Hum Behav 2019 Dec 23;3(12):1319-1331 [FREE Full text] [CrossRef] [Medline]
- Hartmann A, von Wietersheim J, Weiss H, Zeeck A. Patterns of symptom change in major depression: Classification and clustering of long term courses. Psychiatry Res 2018 Sep;267:480-489. [CrossRef] [Medline]
- Zilcha-Mano S, Brown PJ, Roose SP, Cappetta K, Rutherford BR. Optimizing patient expectancy in the pharmacologic treatment of major depressive disorder. Psychol Med 2018 Nov 13;49(14):2414-2420. [CrossRef]
- Kacem A, Hammal Z, Daoudi M, Cohn J. Detecting Depression Severity by Interpretable Representations of Motion Dynamics. Proc Int Conf Autom Face Gesture Recognit 2018 May;2018:739-745 [FREE Full text] [CrossRef] [Medline]
- Bailey N, Hoy K, Rogasch N, Thomson R, McQueen S, Elliot D, et al. Responders to rTMS for depression show increased fronto-midline theta and theta connectivity compared to non-responders. Brain Stimul 2018 Jan;11(1):190-203. [CrossRef] [Medline]
- Browning M, Kingslake J, Dourish CT, Goodwin GM, Harmer CJ, Dawson GR. Predicting treatment response to antidepressant medication using early changes in emotional processing. Eur Neuropsychopharmacol 2019 Jan;29(1):66-75 [FREE Full text] [CrossRef] [Medline]
- Byun S, Kim AY, Jang EH, Kim S, Choi KW, Yu HY, et al. Detection of major depressive disorder from linear and nonlinear heart rate variability features during mental task protocol. Comput Biol Med 2019 Sep;112:103381 [FREE Full text] [CrossRef] [Medline]
- Cash RFH, Cocchi L, Anderson R, Rogachov A, Kucyi A, Barnett AJ, et al. A multivariate neuroimaging biomarker of individual outcome to transcranial magnetic stimulation in depression. Hum Brain Mapp 2019 Nov 01;40(16):4618-4629 [FREE Full text] [CrossRef] [Medline]
- Furukawa TA, Debray TPA, Akechi T, Yamada M, Kato T, Seo M, et al. Can personalized treatment prediction improve the outcomes, compared with the group average approach, in a randomized trial? Developing and validating a multivariable prediction model in a pragmatic megatrial of acute treatment for major depression. Journal of Affective Disorders 2020 Sep;274:690-697. [CrossRef]
- Horigome T, Sumali B, Kitazawa M, Yoshimura M, Liang K, Tazawa Y, et al. Evaluating the severity of depressive symptoms using upper body motion captured by RGB-depth sensors and machine learning in a clinical interview setting: A preliminary study. Compr Psychiatry 2020 Feb 20;98:152169 [FREE Full text] [CrossRef] [Medline]
- Redlich R, Opel N, Grotegerd D, Dohm K, Zaremba D, Bürger C, et al. Prediction of Individual Response to Electroconvulsive Therapy via Machine Learning on Structural Magnetic Resonance Imaging Data. JAMA Psychiatry 2016 Jun 01;73(6):557-564. [CrossRef] [Medline]
- Zhou X, Wang Y, Zeng D. Outcome-Weighted Learning for Personalized Medicine with Multiple Treatment Options. Proc Int Conf Data Sci Adv Anal 2018 Oct;2018:565-574 [FREE Full text] [CrossRef] [Medline]
- Barrett BW, Abraham AG, Dean LT, Plankey MW, Friedman MR, Jacobson LP, et al. Social inequalities contribute to racial/ethnic disparities in depressive symptomology among men who have sex with men. Soc Psychiatry Psychiatr Epidemiol 2021 Feb 11;56(2):259-272 [FREE Full text] [CrossRef] [Medline]
- Chekroud AM, Zotti RJ, Shehzad Z, Gueorguieva R, Johnson MK, Trivedi MH, et al. Cross-trial prediction of treatment outcome in depression: a machine learning approach. The Lancet Psychiatry 2016 Mar;3(3):243-250. [CrossRef]
- Foster S, Mohler-Kuo M, Tay L, Hothorn T, Seibold H. Estimating patient-specific treatment advantages in the 'Treatment for Adolescents with Depression Study'. J Psychiatr Res 2019 May;112:61-70. [CrossRef] [Medline]
- Kambeitz J, Goerigk S, Gattaz W, Falkai P, Benseñor IM, Lotufo PA, et al. Clinical patterns differentially predict response to transcranial direct current stimulation (tDCS) and escitalopram in major depression: A machine learning analysis of the ELECT-TDCS study. J Affect Disord 2020 Mar 15;265:460-467. [CrossRef] [Medline]
- Kessler RC, van Loo HM, Wardenaar KJ, Bossarte RM, Brenner LA, Cai T, et al. Testing a machine-learning algorithm to predict the persistence and severity of major depressive disorder from baseline self-reports. Mol Psychiatry 2016 Oct;21(10):1366-1371 [FREE Full text] [CrossRef] [Medline]
- Pratap A, Atkins DC, Renn BN, Tanana MJ, Mooney SD, Anguera JA, et al. The accuracy of passive phone sensors in predicting daily mood. Depress Anxiety 2019 Jan 21;36(1):72-81 [FREE Full text] [CrossRef] [Medline]
- Rajpurkar P, Yang J, Dass N, Vale V, Keller AS, Irvin J, et al. Evaluation of a Machine Learning Model Based on Pretreatment Symptoms and Electroencephalographic Features to Predict Outcomes of Antidepressant Treatment in Adults With Depression: A Prespecified Secondary Analysis of a Randomized Clinical Trial. JAMA Netw Open 2020 Jun 01;3(6):e206653 [FREE Full text] [CrossRef] [Medline]
- Kautzky A, Möller HJ, Dold M, Bartova L, Seemüller F, Laux G, et al. Combining machine learning algorithms for prediction of antidepressant treatment response. Acta Psychiatr Scand 2021 Jan 27;143(1):36-49 [FREE Full text] [CrossRef] [Medline]
- Lee Y, Mansur RB, Brietzke E, Kapogiannis D, Delgado-Peraza F, Boutilier JJ, et al. Peripheral inflammatory biomarkers define biotypes of bipolar depression. Mol Psychiatry 2021 Jul 03;26(7):3395-3406 [FREE Full text] [CrossRef] [Medline]
- Ma Y, Ji J, Huang Y, Gao H, Li Z, Dong W, et al. Implementing machine learning in bipolar diagnosis in China. Transl Psychiatry 2019 Nov 18;9(1):305 [FREE Full text] [CrossRef] [Medline]
- Deng F, Wang Y, Huang H, Niu M, Zhong S, Zhao L, et al. Abnormal segments of right uncinate fasciculus and left anterior thalamic radiation in major and bipolar depression. Prog Neuropsychopharmacol Biol Psychiatry 2018 Feb 02;81:340-349. [CrossRef] [Medline]
- Nielsen SFV, Madsen KH, Vinberg M, Kessing LV, Siebner HR, Miskowiak KW. Whole-Brain Exploratory Analysis of Functional Task Response Following Erythropoietin Treatment in Mood Disorders: A Supervised Machine Learning Approach. Front Neurosci 2019 Nov 20;13:1246 [FREE Full text] [CrossRef] [Medline]
- Hoogendoorn M, Berger T, Schulz A, Stolz T, Szolovits P. Predicting Social Anxiety Treatment Outcome Based on Therapeutic Email Conversations. IEEE J. Biomed. Health Inform 2017 Sep;21(5):1449-1459. [CrossRef]
- Ihmig FR, Neurohr-Parakenings F, Schäfer SK, Lass-Hennemann J, Michael T. On-line anxiety level detection from biosignals: Machine learning based on a randomized controlled trial with spider-fearful individuals. PLoS One 2020 Jun 23;15(6):e0231517 [FREE Full text] [CrossRef] [Medline]
- Sharma A, Verbeke WJMI. Understanding importance of clinical biomarkers for diagnosis of anxiety disorders using machine learning models. PLoS One 2021 May 10;16(5):e0251365 [FREE Full text] [CrossRef] [Medline]
- Demiris G, Corey Magan KL, Parker Oliver D, Washington KT, Chadwick C, Voigt JD, et al. Spoken words as biomarkers: using machine learning to gain insight into communication as a predictor of anxiety. J Am Med Inform Assoc 2020 Jun 01;27(6):929-933 [FREE Full text] [CrossRef] [Medline]
- Frick A, Engman J, Alaie I, Björkstrand J, Gingnell M, Larsson E, et al. Neuroimaging, genetic, clinical, and demographic predictors of treatment response in patients with social anxiety disorder. J Affect Disord 2020 Jan 15;261:230-237 [FREE Full text] [CrossRef] [Medline]
- Lebowitz ER, Zilcha-Mano S, Orbach M, Shimshoni Y, Silverman WK. Moderators of response to child-based and parent-based child anxiety treatment: a machine learning-based analysis. J Child Psychol Psychiatry 2021 Oct 24;62(10):1175-1182. [CrossRef] [Medline]
- Lenhard F, Sauer S, Andersson E, Månsson KN, Mataix-Cols D, Rück C, et al. Prediction of outcome in internet-delivered cognitive behaviour therapy for paediatric obsessive-compulsive disorder: A machine learning approach. Int J Methods Psychiatr Res 2018 Mar 28;27(1):e1576 [FREE Full text] [CrossRef] [Medline]
- Schultebraucks K, Qian M, Abu-Amara D, Dean K, Laska E, Siegel C, et al. Pre-deployment risk factors for PTSD in active-duty personnel deployed to Afghanistan: a machine-learning approach for analyzing multivariate predictors. Mol Psychiatry 2021 Sep 02;26(9):5011-5022 [FREE Full text] [CrossRef] [Medline]
- Rangaprakash D, Deshpande G, Daniel TA, Goodman AM, Robinson JL, Salibi N, et al. Compromised hippocampus-striatum pathway as a potential imaging biomarker of mild-traumatic brain injury and posttraumatic stress disorder. Hum Brain Mapp 2017 Jun 15;38(6):2843-2864 [FREE Full text] [CrossRef] [Medline]
- Schultebraucks K, Shalev AY, Michopoulos V, Grudzen CR, Shin S, Stevens JS, et al. A validated predictive algorithm of post-traumatic stress course following emergency department admission after a traumatic stressor. Nat Med 2020 Jul 06;26(7):1084-1088. [CrossRef] [Medline]
- Grisanzio KA, Goldstein-Piekarski AN, Wang MY, Rashed Ahmed AP, Samara Z, Williams LM. Transdiagnostic Symptom Clusters and Associations With Brain, Behavior, and Daily Function in Mood, Anxiety, and Trauma Disorders. JAMA Psychiatry 2018 Feb 01;75(2):201-209 [FREE Full text] [CrossRef] [Medline]
- Hinrichs R, van Rooij SJH, Michopoulos V, Schultebraucks K, Winters S, Maples-Keller J, et al. Increased Skin Conductance Response in the Immediate Aftermath of Trauma Predicts PTSD Risk. Chronic Stress (Thousand Oaks) 2019 Apr 24;3:247054701984444 [FREE Full text] [CrossRef] [Medline]
- Breen MS, Thomas KG, Baldwin DS, Lipinska G. Modelling PTSD diagnosis using sleep, memory, and adrenergic metabolites: An exploratory machine-learning study. Hum Psychopharmacol 2019 Mar 22;34(2):e2691. [CrossRef] [Medline]
- Jin C, Jia H, Lanka P, Rangaprakash D, Li L, Liu T, et al. Dynamic brain connectivity is a better predictor of PTSD than static connectivity. Hum Brain Mapp 2017 Sep 12;38(9):4479-4496 [FREE Full text] [CrossRef] [Medline]
- Salminen LE, Morey RA, Riedel BC, Jahanshad N, Dennis EL, Thompson PM. Adaptive Identification of Cortical and Subcortical Imaging Markers of Early Life Stress and Posttraumatic Stress Disorder. J Neuroimaging 2019 May;29(3):335-343 [FREE Full text] [CrossRef] [Medline]
- Yuan M, Qiu C, Meng Y, Ren Z, Yuan C, Li Y, et al. Pre-treatment Resting-State Functional MR Imaging Predicts the Long-Term Clinical Outcome After Short-Term Paroxtine Treatment in Post-traumatic Stress Disorder. Front Psychiatry 2018 Oct 30;9:532 [FREE Full text] [CrossRef] [Medline]
- Zandvakili A, Swearingen HR, Philip NS. Changes in functional connectivity after theta-burst transcranial magnetic stimulation for post-traumatic stress disorder: a machine-learning study. Eur Arch Psychiatry Clin Neurosci 2021 Feb 27;271(1):29-37. [CrossRef] [Medline]
- Marmar CR, Brown AD, Qian M, Laska E, Siegel C, Li M, et al. Speech-based markers for posttraumatic stress disorder in US veterans. Depress Anxiety 2019 Jul 22;36(7):607-616 [FREE Full text] [CrossRef] [Medline]
- Iceta S, Tardieu S, Nazare J, Dougkas A, Robert M, Disse E. An artificial intelligence-derived tool proposal to ease disordered eating screening in people with obesity. Eat Weight Disord 2021 Oct 02;26(7):2381-2385. [CrossRef] [Medline]
- Senger K, Schröder A, Kleinstäuber M, Rubel JA, Rief W, Heider J. Predicting optimal treatment outcomes using the Personalized Advantage Index for patients with persistent somatic symptoms. Psychother Res 2022 Feb 29;32(2):165-178. [CrossRef] [Medline]
- Bae S, Chung T, Ferreira D, Dey AK, Suffoletto B. Mobile phone sensors and supervised machine learning to identify alcohol use events in young adults: Implications for just-in-time adaptive interventions. Addict Behav 2018 Aug;83:42-47 [FREE Full text] [CrossRef] [Medline]
- Joseph JE, Vaughan BK, Camp CC, Baker NL, Sherman BJ, Moran-Santa Maria M, et al. Oxytocin-Induced Changes in Intrinsic Network Connectivity in Cocaine Use Disorder: Modulation by Gender, Childhood Trauma, and Years of Use. Front Psychiatry 2019 Jul 19;10:502 [FREE Full text] [CrossRef] [Medline]
- Laska EM, Siegel CE, Lin Z, Bogenschutz M, Marmar CR. Gabapentin Enacarbil Extended-Release Versus Placebo: A Likely Responder Reanalysis of a Randomized Clinical Trial. Alcohol Clin Exp Res 2020 Sep 31;44(9):1875-1884 [FREE Full text] [CrossRef] [Medline]
- Pan Y, Liu H, Metsch LR, Feaster DJ. Factors Associated with HIV Testing Among Participants from Substance Use Disorder Treatment Programs in the US: A Machine Learning Approach. AIDS Behav 2017 Feb 8;21(2):534-546 [FREE Full text] [CrossRef] [Medline]
- Schmitgen MM, Niedtfeld I, Schmitt R, Mancke F, Winter D, Schmahl C, et al. Individualized treatment response prediction of dialectical behavior therapy for borderline personality disorder using multimodal magnetic resonance imaging. Brain Behav 2019 Sep 14;9(9):e01384 [FREE Full text] [CrossRef] [Medline]
- Bang S, Son S, Roh H, Lee J, Bae S, Lee K, et al. Quad-phased data mining modeling for dementia diagnosis. BMC Med Inform Decis Mak 2017 May 18;17(Suppl 1):60-10 [FREE Full text] [CrossRef] [Medline]
- Yao T, Sweeney E, Nagorski J, Shulman JM, Allen GI. Quantifying cognitive resilience in Alzheimer's Disease: The Alzheimer's Disease Cognitive Resilience Score. PLoS One 2020 Nov 5;15(11):e0241707 [FREE Full text] [CrossRef] [Medline]
- Grassi M, Rouleaux N, Caldirola D, Loewenstein D, Schruers K, Perna G, Alzheimer's Disease Neuroimaging Initiative. A Novel Ensemble-Based Machine Learning Algorithm to Predict the Conversion From Mild Cognitive Impairment to Alzheimer's Disease Using Socio-Demographic Characteristics, Clinical Information, and Neuropsychological Measures. Front Neurol 2019 Jul 16;10:756 [FREE Full text] [CrossRef] [Medline]
- Li Q, Zhao Y, Chen Y, Yue J, Xiong Y. Developing a machine learning model to identify delirium risk in geriatric internal medicine inpatients. Eur Geriatr Med 2022 Feb 23;13(1):173-183. [CrossRef] [Medline]
- Andersson S, Bathula DR, Iliadis SI, Walter M, Skalkidou A. Predicting women with depressive symptoms postpartum with machine learning methods. Sci Rep 2021 Apr 12;11(1):7877 [FREE Full text] [CrossRef] [Medline]
- Li Y, Rosenfeld B, Pessin H, Breitbart W. Bayesian Nonparametric Clustering of Patients with Advanced Cancer on Anxiety and Depression. 2017 Presented at: 16th IEEE International Conference on Machine Learning and Applications (ICMLA); December 18-21, 2017; Cancun, Mexico p. 674-678. [CrossRef]
- Zhou S, Fei S, Han H, Li J, Yang S, Zhao C. A Prediction Model for Cognitive Impairment Risk in Colorectal Cancer after Chemotherapy Treatment. Biomed Res Int 2021 Feb 20;2021:6666453-6666413 [FREE Full text] [CrossRef] [Medline]
- Vitinius F, Escherich S, Deter H, Hellmich M, Jünger J, Petrowski K, et al. Somatic and sociodemographic predictors of depression outcome among depressed patients with coronary artery disease - a secondary analysis of the SPIRR-CAD study. BMC Psychiatry 2019 Feb 04;19(1):57 [FREE Full text] [CrossRef] [Medline]
- Wallert J, Gustafson E, Held C, Madison G, Norlund F, von Essen L, et al. Predicting Adherence to Internet-Delivered Psychotherapy for Symptoms of Depression and Anxiety After Myocardial Infarction: Machine Learning Insights From the U-CARE Heart Randomized Controlled Trial. J Med Internet Res 2018 Oct 10;20(10):e10754 [FREE Full text] [CrossRef] [Medline]
- Gu S, Zhou J, Yuan C, Ye Q. Personalized prediction of depression in patients with newly diagnosed Parkinson's disease: A prospective cohort study. J Affect Disord 2020 May 01;268:118-126. [CrossRef] [Medline]
- Montazeri F, de Bildt A, Dekker V, Anderson GM. Network Analysis of Behaviors in the Depression and Autism Realms: Inter-Relationships and Clinical Implications. J Autism Dev Disord 2020 May 18;50(5):1580-1595. [CrossRef] [Medline]
- Ekhtiari H, Kuplicki R, Yeh H, Paulus MP. Physical characteristics not psychological state or trait characteristics predict motion during resting state fMRI. Sci Rep 2019 Jan 23;9(1):419 [FREE Full text] [CrossRef] [Medline]
- Rosellini AJ, Stein MB, Benedek DM, Bliese PD, Chiu WT, Hwang I, et al. Predeployment predictors of psychiatric disorder-symptoms and interpersonal violence during combat deployment. Depress Anxiety 2018 Nov 13;35(11):1073-1080 [FREE Full text] [CrossRef] [Medline]
- Smith R, Feinstein JS, Kuplicki R, Forthman KL, Stewart JL, Paulus MP, Tulsa 1000 Investigators, et al. Perceptual insensitivity to the modulation of interoceptive signals in depression, anxiety, and substance use disorders. Sci Rep 2021 Jan 22;11(1):2108 [FREE Full text] [CrossRef] [Medline]
- Liang S, Vega R, Kong X, Deng W, Wang Q, Ma X, et al. Neurocognitive Graphs of First-Episode Schizophrenia and Major Depression Based on Cognitive Features. Neurosci Bull 2018 Apr 2;34(2):312-320 [FREE Full text] [CrossRef] [Medline]
- Popovic D, Ruef A, Dwyer DB, Antonucci LA, Eder J, Sanfelici R, PRONIA Consortium. Traces of Trauma: A Multivariate Pattern Analysis of Childhood Trauma, Brain Structure, and Clinical Phenotypes. Biol Psychiatry 2020 Dec 01;88(11):829-842. [CrossRef] [Medline]
- Zandvakili A, Philip NS, Jones SR, Tyrka AR, Greenberg BD, Carpenter LL. Use of machine learning in predicting clinical response to transcranial magnetic stimulation in comorbid posttraumatic stress disorder and major depression: A resting state electroencephalography study. J Affect Disord 2019 Jun 01;252:47-54 [FREE Full text] [CrossRef] [Medline]
- Perez Arribas I, Goodwin GM, Geddes JR, Lyons T, Saunders KEA. A signature-based machine learning model for distinguishing bipolar disorder and borderline personality disorder. Transl Psychiatry 2018 Dec 13;8(1):274 [FREE Full text] [CrossRef] [Medline]
- Tate AE, McCabe RC, Larsson H, Lundström S, Lichtenstein P, Kuja-Halkola R. Predicting mental health problems in adolescence using machine learning techniques. PLoS One 2020 Apr 6;15(4):e0230389 [FREE Full text] [CrossRef] [Medline]
- Liu X, Rivera SC, Moher D, Calvert MJ, Denniston AK, SPIRIT-AICONSORT-AI Working Group. Reporting guidelines for clinical trial reports for interventions involving artificial intelligence: the CONSORT-AI Extension. BMJ 2020 Sep 09;370:m3164 [FREE Full text] [CrossRef] [Medline]
- Rivera SC, Liu X, Chan A, Denniston AK, Calvert MJ, SPIRIT-AICONSORT-AI Working Group. Guidelines for clinical trial protocols for interventions involving artificial intelligence: the SPIRIT-AI Extension. BMJ 2020 Sep 09;370:m3210 [FREE Full text] [CrossRef] [Medline]
- Norgeot B, Quer G, Beaulieu-Jones BK, Torkamani A, Dias R, Gianfrancesco M, et al. Minimum information about clinical artificial intelligence modeling: the MI-CLAIM checklist. Nat Med 2020 Sep 09;26(9):1320-1324 [FREE Full text] [CrossRef] [Medline]
- Collins GS, Dhiman P, Andaur Navarro CL, Ma J, Hooft L, Reitsma JB, et al. Protocol for development of a reporting guideline (TRIPOD-AI) and risk of bias tool (PROBAST-AI) for diagnostic and prognostic prediction model studies based on artificial intelligence. BMJ Open 2021 Jul 09;11(7):e048008 [FREE Full text] [CrossRef] [Medline]
- Altman DG, Simera I. A history of the evolution of guidelines for reporting medical research: the long road to the EQUATOR Network. J R Soc Med 2016 Feb;109(2):67-77 [FREE Full text] [CrossRef] [Medline]